Let’s say you’re hosting a dinner party and must decide between spaghetti and tacos. A quick vote settles the issue—easy, right? Now, imagine you want to test whether garlic bread or salad pairs better with spaghetti or if salsa or guacamole steals the show with the tacos.
Suddenly, your testing and experimenting get a lot more complex with multiple combination possibilities.
This is the difference between A/B and multivariate testing. A/B is like choosing between two dishes—simple and focused. Multivariate goes deeper, testing how combinations of elements work together.
This article will explore when to use each method to help you make smarter, data-driven decisions. Let’s find out!
Understanding Multivariate Testing vs A/B Testing
Both A/B testing and multivariate testing are popular methods for testing hypotheses in marketing, web design, product development, and user experience optimization.
While both help you improve performance through data-driven decision-making, they differ in complexity, scope, and applicability.
What is A/B Testing?
A/B testing, or split testing, is a simple method for comparing two versions of a single variable to determine which performs better. You can use it to test a hypothesis about a single element on a webpage, app, or marketing campaign.
For example, a company might want to compare the effectiveness of two different call-to-action (CTA) button colors on a landing page.
Airbnb regularly uses A/B testing to optimize its website and app interfaces.
In 2018, the holiday rental company used A/B testing to optimize a pricing feature that offered hosts personalized price suggestions based on demand, competition, and location. The test revealed higher booking rates for hosts using the feature, leading to its global rollout.
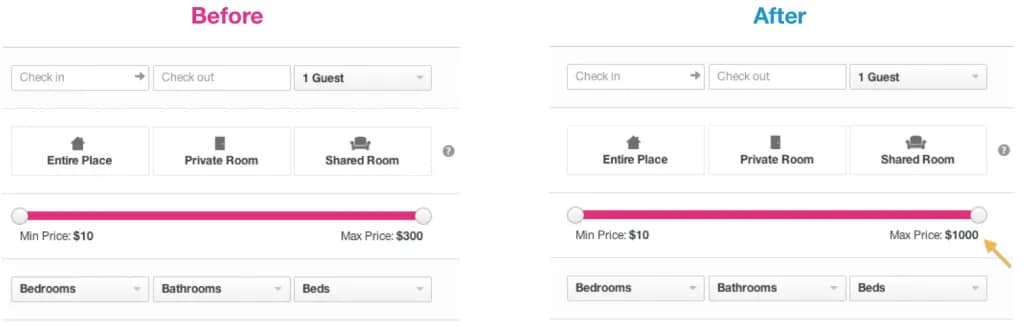
Key advantages of A/B testing:
- Simplicity: A/B testing is straightforward to set up and analyze.
- Clear results: With only one variable being tested, results are easy to interpret.
- Low traffic requirements: A/B tests can be effective even with a lower traffic volume.
What is Multivariate Testing?
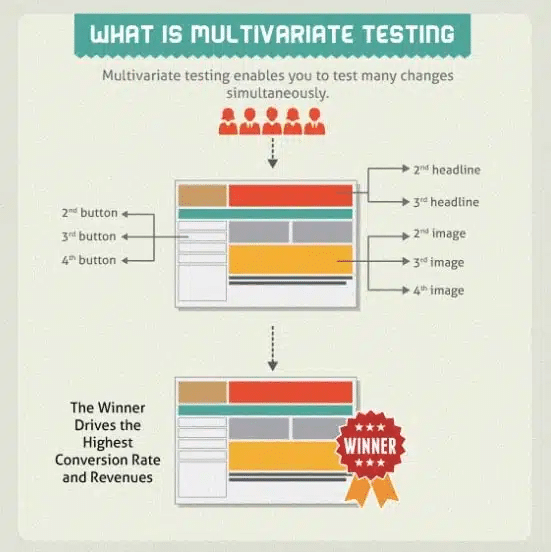
Multivariate testing (MVT) takes A/B testing a step further by testing multiple variables simultaneously. Instead of testing just one change, MVT tests combinations of changes across multiple elements of a webpage or marketing campaign. The goal is to understand how different combinations of elements impact the overall performance.
For example, a business could simultaneously test different combinations of headline text, images, and button designs to determine which combination delivers the highest conversion rate.
Key advantages of multivariate testing:
- Comprehensive analysis: MVT allows businesses to test a range of variations simultaneously, offering a deeper understanding of the interactions between different elements.
- Efficiency with high traffic: MVT is ideal for websites or platforms with high traffic; the more data you have, the more reliable the results are.
- Better insights: MVT can show how different elements interact with each other, revealing hidden opportunities for optimization that A/B testing alone might miss.
Difference Between Multivariate Testing vs. A/B Testing
To truly determine which testing tools to use, you must first discover their differences. Here’s an overview of the common differences between multivariate and A/B testing.
- Scope of testing: This refers to the number and types of elements being tested in an experiment and how broad or narrow the focus of the test is.
- A/B testing: Focuses on testing a single element, such as a button color or headline copy.
- Multivariate testing Tests multiple elements simultaneously, such as the headline, button color, and image placement, to understand how combinations of changes affect performance.
- Complexity:
It refers to the ease or difficulty of designing, implementing, and analyzing the test.
- A/B Testing: Simpler to set up and analyze, especially for beginners. For instance, changing the text of a call-to-action button and comparing its performance with the original button is straightforward to implement and evaluate. It’s ideal for beginners or teams with limited resources.
- Multivariate testing: More complex due to the more variations and combinations involved. For example, if you’re testing three headlines, three button colors, and two images, there are 18 combinations to analyze. This requires advanced tools and statistical analysis to determine which combinations are most effective.
- Traffic requirements:
It refers to the amount of user activity (e.g., website visits, clicks, or impressions) needed to achieve statistically significant results.
- A/B testing: Works well with lower traffic volumes, as you only test one variation at a time. For instance, if you have 1,000 visitors to your website daily, you can run an A/B test and get reliable results relatively quickly since only two groups are being compared.
- Multivariate testing: Requires a higher traffic volume to ensure that the test reaches statistical significance for each variation and combination. Let’s say you’re testing four combinations, each needing at least 1,000 visitors. In that case, you’ll require at least 4,000 visitors to gather meaningful insights. This makes MVT better suited for high-traffic websites or apps.
- Result analysis:
This entails the process of interpreting the data collected during the test and understanding which variation or combination performed best.
- A/B testing: Results are usually clear-cut because only one variable is being tested. For example, if Version A of your landing page converts at 20% and Version B converts at 25%, you can quickly identify the winner.
- Multivariate testing: Results require more advanced analysis to understand which combinations of elements are most effective. For instance, if you’re testing multiple elements, you’ll need to understand the individual performance of each element and how they interact. In this case, you’ll need specialized software and statistical models to analyze the results accurately.
- Duration:
Here, duration refers to how long a test needs to run to collect enough data for statistically significant results.
- A/B testing: Typically faster, as it tests fewer combinations. For instance, an A/B test with two versions of an email subject line might take a few days to conclude.
- Multivariate testing: Due to its complexity and the number of variations, it may take longer to reach conclusions. For instance, testing multiple variations on a homepage might take weeks or even months, depending on the traffic volume and the number of combinations being analyzed.
When to Use A/B Testing Tools vs. Multivariate Testing Tools
Now that you know the differences, it’s time to choose an option to run your experiments. Choosing between A/B testing and multivariate testing tools depends on your goals, resources, traffic, and the complexity of the changes you want to test. Here’s a closer look at when and why you’d use each testing tool.
When to Use A/B Testing Tools
A/B testing is like a “trial by duel”—you pit two versions of a single element against each other and see which one wins. It’s straightforward, quick, and ideal for specific use cases.
- Simple scenarios: A/B testing is perfect when you want to simultaneously test one variable or change. Examples include:
- Testing the color of a CTA button (e.g., red vs. green) to see which gets more clicks.
- Comparing two different headlines for an email campaign.
- When there’s limited traffic: A/B testing is the better option for businesses with low website traffic. Because it only compares two variations, it requires fewer visitors to reach statistically significant results.
When you need quick and precise results: A/B tests work well when you need quick and actionable results. Since you’re testing just one change, it’s easy to interpret the outcome and implement the winning variation.
For example, during the 2008 U.S. presidential election, Obama’s campaign used A/B testing to optimize donation pages and email subject lines for quick, precise results. Simple changes like testing headlines, images, and workflows boosted engagement, added 2.88 million email signups, and generated $60 million in donations, showing how A/B testing comes in handy when you want outcomes quickly and clearly.
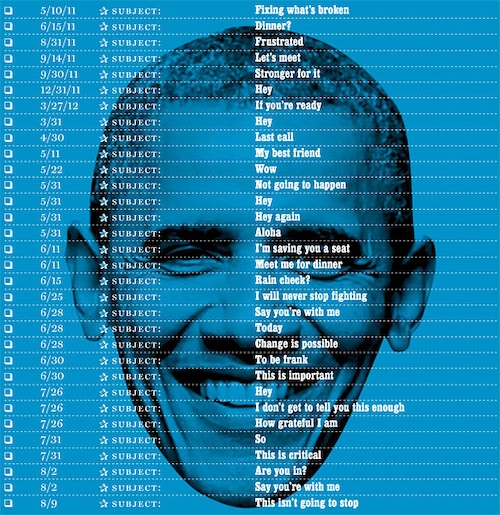
- You require low complexity in the test setup: If your team is new to testing or doesn’t have advanced analytics expertise, A/B testing is easier to set up and manage. You don’t need sophisticated web analytics tools or data science knowledge—just a hypothesis and a clear goal.
Now, let’s see the other side.
When to Use Multivariate Testing Tools
Multivariate testing, on the other hand, is like playing chess—it involves evaluating multiple web page elements simultaneously and understanding how they interact. This approach is suited for more complex scenarios.
- Complex scenarios: If you’re making several changes simultaneously (e.g., modifying headlines, images, and button colors), multivariate testing is the right choice. It helps you determine which combination of elements works best.
Pro tip: Use multivariate testing when you suspect that two or more elements on a page interact with each other. For example, a headline and an image might work well together, but they might not perform as effectively separately.
- High-traffic websites: Multivariate testing requires a large traffic volume to split users across all the variations and still produce statistically significant results. It’s a numbers game—the more variations, the more visitors you need.
- When trying to understand the interaction between multiple variants or elements: One of the most significant advantages of multivariate testing is that it reveals how different elements interact. For example:
- A CTA button might perform well with one headline but poorly with another.
- Testing combinations of layout, color scheme, and content placement can uncover synergies that A/B testing can’t.
Over to You!
Both A/B and multivariate testing methods have their strengths, but choosing the right tool depends on the scenario, traffic volume, complexity, and long-term goals.
A/B testing is a practical choice for businesses looking for quick wins. For those with the resources and traffic to handle complex analyses, multivariate testing offers invaluable insights into how multiple changes interact.